
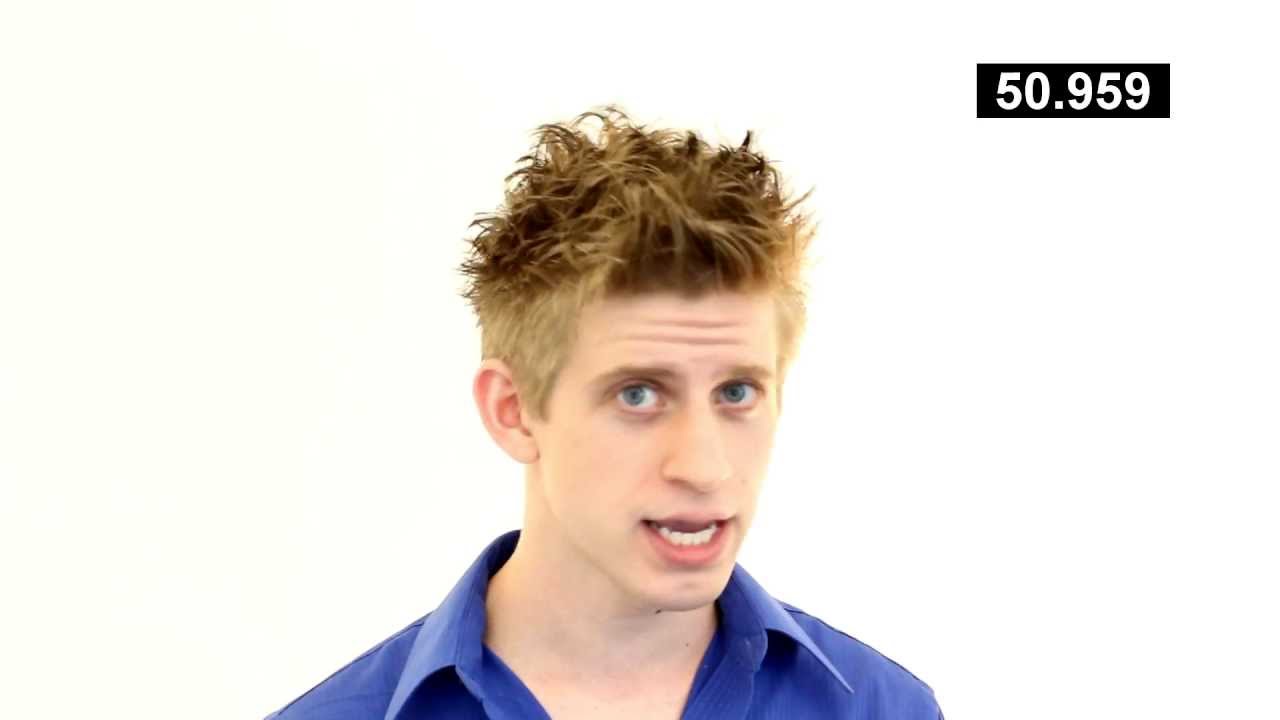
The best baselines to study this work would have been VCL and likes. Could the authors point out why using model parameters as training data for VAE (like they did) is better than standard VAE training in a continual setting? It seems like a lot of machinery has been used in this work without properly grounding the study in the literature. In this work and in the VCL type approaches the objective is to model the distribution over network parameters. Barring Bayesian continual learning, the paper is well-grounded in the recent literature.ġ) Why not posterior over model parameters: It’s not clear to me what is the advantage of this framework over standard variational continual learning type approaches (,, etc). Although the method seems overly complicated (more on this in the negative section) but the overall writing of the paper is very good. By and large, the paper is well-written. I am not giving a clear accept because I still believe that the method is unnecessarily cumbersome and some of the components can be simplified. Therefore, I am increasing my score to marginally above acceptance threshold. Anyhow, the rebuttal is strong and addressed most of my concerns. I don't agree with the author's' assertion that VCL does not learn a distribution over model parameters. While I still believe that Bayesian Continual Learning type baselines would be better suitable for this work and I encourage the authors to add those in their final draft, comparison with CN-DPM, if done correctly, suggests that MERLIN can outperform other Bayesian baselines (although I am not sure if the authors used CN-DPM correctly or in the right setting). The authors adequately addressed some of my concerns.

The experiments are reported on the standard continual learning benchmarks for image classification. This set is then fine-tuned on the replay buffer and the results are ensembled over the set. At inference time, the latent is sampled from the task-specific prior or all the priors (depending on whether the task information is present or not), ‘E’ number of models set is sampled from the decoder. After training each task, the updated VAE is consolidated for previous tasks by sampling from the task-specific learned priors, generating the parameters from those samples, and updating all the VAE parameters using those generated samples as supervisory signals. One notable change is that the (parametric) prior over the latent distribution is task-specific and is learned along with the VAE parameters. The standard VAE ELBO is maximized during the training.
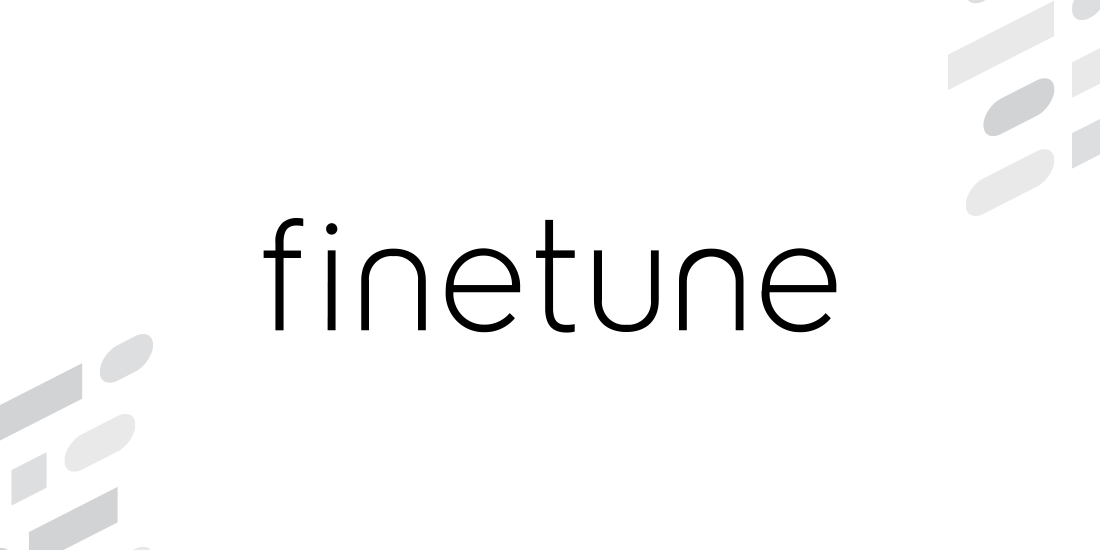
A VAE is then trained, using these ‘B’ model parameters as training points to learn an encoder (mapping the parameters to the latent) and decoder (mapping the latent to model parameters). More specifically, given a dataset of a task t, the idea is to train ‘B’ separate models.
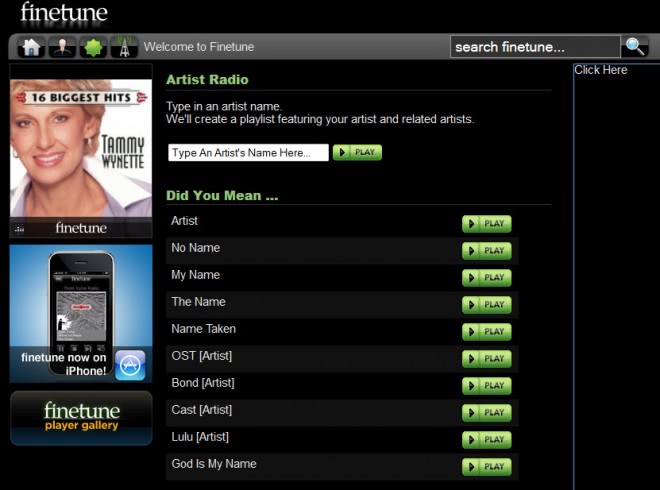
VAE is used to model the distribution over the model parameters. Summary and Contributions: The paper proposes an online continual learning method, MERLIN, that learns a distribution over the task-specific model parameters given a context (task identifiers, etc). Review for NeurIPS paper: Meta-Consolidation for Continual Learning NeurIPS 2020 Meta-Consolidation for Continual Learning
